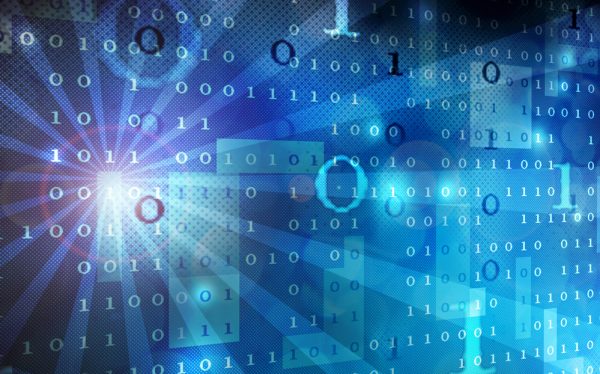
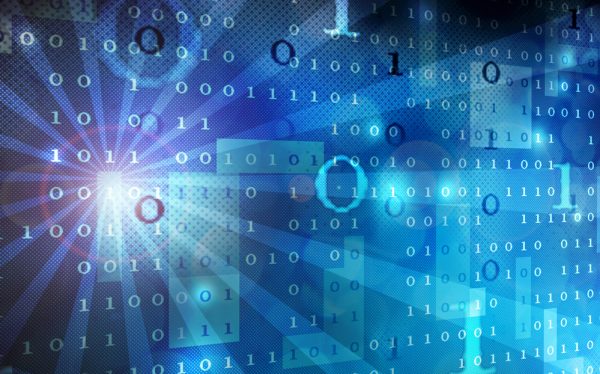
Data & Analytics Strategy
by Tom Dissing
As data and analytics transform industries at a continuously faster pace, the strategies of leading companies offer a road map for success
Forty-seven percent of companies say that data and analytics have fundamentally changed the nature of competition in their industries in the past three years. Traditional competitors are 2.5x more likely to launch new data and analytics businesses, pooling data via a shared utility and forming data-related partnerships along the value chain (Note 1). Companies see the potential to reduce total annual costs by 33% and increase incremental revenue by 31% from using data they already have. Add those two numbers together, and the incentive to monetise data is clear: It could vastly boost the bottom line (Note 2).
Yet, despite these competitive changes and tangible financial opportunities, 4 in 10 companies say that their company is only responding in an ad hoc manner (Note 1). With 86% of businesses saying 2019 is the year in which they will extract value from data. The time to hesitate is over. The race is on!
Barriers to Success
What are the challenges companies are facing to successfully implementing data and analytics initiatives? As illustrated in Figure 1, the two main barriers are lack of strategy and lack of architecture and technology infrastructure, with a lack of strategy increasing in importance as results requires a strategically coherent approach.
Figure 1: Barriers to Success
Source: McKinsey (Note 1)
These two barriers as well as ensuring senior management leadership of data and analytics efforts are also the key contributors to success. So, what can companies do to overcome these barriers and start to leverage them to become data-driven?
Data & Analytics Strategy
A comprehensive strategy is, of course, critical to success in nearly any business endeavour, and data and analytics initiatives are no different. Leading companies are creating data and analytics strategies.
A data strategy helps companies clarify the primary purpose of their data and guides them in strategic data management. Generally, there are three main strategic purposes of a data strategy:
- Increase revenue
- Improve operational efficiencies (e.g. cost, time and resource reductions)
- Monetising data (e.g. Weatherzone or FitBit selling their data to other companies)
Australian companies are primarily using data to generate revenue growth and cost efficiencies, whereas monetising data is viewed as a potential next level of sophistication and opportunities.
A data strategy should consider both the internal strategic drivers as well as the external environment. The latter being explored via customer research, industry trends, technology and data trends and leading data and analytics practices.
A data strategy should be focused on the target state and derived by considering the following:
- Vision & Outcomes
- Strategic Alignment
- Customer & User Journeys
- Capabilities & Architecture
- Accelerators and Risks
The target state will require changes to the operating model, that should include the following five elements:
- Organisation
- Data & Processes
- Systems & Tools
- Engagement
- Governance
For insights into how to design a new operating model, refer to Technology Connect’s Operating Model Canvas as set out in the Next Generation IT Operating Model insights paper.
Finally, the data strategy needs an Execution Plan, which typically contains an Investment Case for the strategy, i.e. the costs and benefits over the strategic time horizon, and a detailed road map to ensure all components of the target state are sequenced correctly and allocated to key stakeholders proving the shortest path the highest value.
Data & Analytics Architecture and Technology Infrastructure
To execute the data and analytics strategy organisations typically need to invest in the deployment of a modern and scalable data architecture.
Cloud data warehouse solutions enable compute resources to be scaled with no effective limit. This enables dynamically spinning up new clusters, pay as you go (by the second) and simplifies capacity planning. There are plenty of Software as a Service (SaaS) data warehouse solutions available. A leading example is Snowflake, that also facilitates workload separation which solves the problem of multiple teams competing for shared fixed resources to run data queries. In other words, it reduces the time it takes to query the data warehouse for multiple teams. Essentially, Data Warehouse as a Service (DWaaS) enable organisations to quickly deploy a data warehouse prototype, including leveraging inbuilt and continuously updated workflow automation and security features. DWaaS also facilitates data sharing to and from multiple sources in a data eco-system, which traditionally has been a very expensive and time-consuming undertaking for many big data and analytics teams.
From an analytics perspective, there are several solutions that, when combined with a cloud-based data warehouse solution, can enable the insights for faster and better revenue growth and operational efficiency decisions. Solutions such as Qlik Sense and Tableau (now part of Salesforce) are examples of leading analytics solutions. Qlik’s recent acquisition of Attunity, a leading provider of data integration and big data management solutions, makes this a very exciting solution for a simplified, but modern architecture.
There are other components of a modern data and analytics architecture that will enhance the data and analytics capabilities, e.g. Alteryx to automate the data warehouse queries and data extracts and DataRobot to automate reporting to drive user self-sufficiency.
Creating a Data-driven Culture
In conjunction with a data and analytics strategy, leading companies are making data a core part of employee collaboration, engagements and mindsets by educating them as part of a broader effort to build a strong data-driven culture.
If you haven’t yet got a data and analytics strategy, it can be as simple as creating three to five use cases for how data that you already have can improve your operational efficiency. This should be followed by a prototype to incorporating your use cases. The prototype will allow you to build momentum and prove the tangible benefits of being a data-driven organisation.
Notes:
- McKinsey: Catch them if you can: How leaders in data and analytics have pulled ahead, 2019
- PwC Data Trust Pulse Survey, 2019
If you are interested in an assessment of your company’s Data & Analytics Pulse as an input into developing your Data & Analytics Strategy or compare your organisation to peers, then click on the following link to a secure Microsoft Form Survey. The survey takes approx. 10 minutes to complete. Your response will remain confidential: Data & Analytics Pulse Survey
For additional insights and ideas: technologyconnect.com.au
or follow us on LinkedIn via: linkedin.com/company/technologyconnect
Tom Dissing is the founder and Managing Director of Technology Connect. He is a certified Data & Analytics Master with deep expertise in guiding medium and large organisations on their digital transformation journeys as they re-imagine and scale their businesses in response to the disruptive challenges and opportunities of the 4th Industrial Revolution. He has advised senior executives in Financial Services (Banking and Insurance), Media & Entertainment, Construction & Engineering, Technology Services and Government (Federal and State) in Australia, New Zealand, Asia and Europe.
Copyright © 2019 Technology Connect. All rights reserved.